The Evolution of Data Science in the Recent Years
In recent years, significant advancements have been attained in the field of Information and Technology (IT). Some of the tech ecosystem’s innovative features are truly commendable. Analytics and data have been the most widely used words of the last two decades. As such, it is crucial to know why they are interconnected, what roles are currently evolving in the industry, and how companies are being reshaped. To achieve this understanding, it’s critical to Learn Data Science and its evolution.
One outcome of Data Science evolution was a gradual change to increasingly conservative programming. Besides, it was discovered that while simpler ones work more efficiently, data scientists will invest so much energy and time into designing extremely complex algorithms.
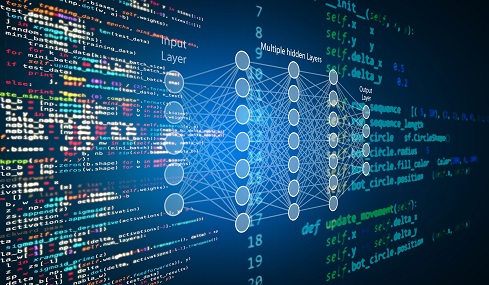
Also viewed as a blessing to those who are already knowledgeable of its potential, technology may also be a threat to viewers who cannot catch up with its rapid growth. Every era has had its revolutionary moments and an equal proportion of victims. Each currency-driven industry now relies entirely on Data and Analytics for its sustainability. This article attempts to look through various analytical stages: to summarize the multiple phases, to narrate situations that have never been clarified, and to keep a close eye on the path that awaits.
Analytics 0.1 – Need for Business Intelligence
This was a data warehouse uprising in which customers (businesses) and industrial processes (transactions) became merged into a big repository such as eCDW (Enterprise Consolidated Data Warehouse). Significant progress has been made in obtaining an objective and in-depth understanding of critical financial trends – thus giving managers the empirical knowledge to go beyond emotion when making decisions.
The eCDW data was captured, processed, and queried by utilizing the ETL & BI tools. During this process, the type of analytics used was primarily categorized as Descriptive and Diagnostic.
Nevertheless, the critical limitations found during this period were that potential data resources were only used inside organizations. This means that business intelligence practices discussed only what had happened previously and gave no forecasts of its future trends.
Analytics 0.2 – Big Data
The previous era’s disadvantages became more popular by the day as businesses moved away from their comfort zone and started taking a broader approach towards obtaining a sophisticated context of analytics. Surprisingly, customers reacted very well to the new strategy and requested information from outside. What businesses needed from their workers was to support engineering systems with a fast processing engine to handle large volumes of data.
With the community’s unparalleled support, Positions such as Big-Data Engineers & Hadoop Administrators have evolved in the job sector and have become vital to any IT organization. Tech companies raced to create new systems that could not only absorb, transform, and process big data around eCDW / Data Lakes but also incorporate predictive analytics above it.
It uses concise and predictive analytics results to identify patterns, clusters, and exceptions, and predict potential trends, making it a valuable resource for forecasting.
Analytics 3.0 – The Data Enriched Offerings
Data analytics leading companies started evaluating goods and applications to support customer-facing services. With improved search algorithms, suggestions, and recommendations for products to purchase, and highly targeted advertising, all powered by analytics embedded in massive quantities of data, they attracted visitors to their platforms. The Big-Data-phenomena outbreak spread fast. The broad adoption of big data systems, on the other hand, had a mixed effect. While the tech-savvy giants advanced by making lots of money, many other businesses and non-tech companies suffered miserably at the cost of not understanding the details. As a result, a Data Science area of study was developed that used experimental methods, exploratory techniques, algorithms, and systems to derive information and insights from data in different types.
The technology industry expanded with the advantages of applying Data Science strategies, exploiting the full power of prescriptive and predictive analytics, i.e., avoiding a potential issue, or taking full advantage of a strong trend. Companies began relying on analytics not just in the conventional sense by enhancing internal business decisions but also through creating more valuable products. This was the essence of analytics 0.3.
Analytics 4.0 – The Automated Capabilities
Four forms of analytics have always existed: descriptive, reporting on the past, diagnostic, using past data to analyze the present, predictive, using observations based on previous data to forecast the future, and prescriptive, using models to determine optimal attitudes and actions. However, in a broad sense, Analytics 3.0 contains all of the above styles. It emphasizes the last.
Analytics 3.0 offers industrial strength and the ability to scale decision-making processes. By generating even more models via machine learning, such as ML forecasting methodology, an organization’s forecasts will become even more granular and accurate. Having said that, cost and time to deploy these custom models were not entirely feasible, and a cheaper and faster approach was needed. Eventually, there came the need for optimization by intelligent systems, and this concept that appeared on the horizon was when Analytics 4.0 came in.
The data included here emerged from vast heterogeneous sources of indigenous types — one requiring sophisticated training methods — and particularly those that can maintain (make suggestions, enhance decision-making, take effective action) themselves. Within this era, the use of software-mining techniques and artificial intelligence algorithms, along with the current descriptive-predictive-prescriptive analysis, comes to complete fruit. This is another reason why Automated Analytics is considered the next analytical maturity level.
Analytics 5.0 – Future of Analytics
Analytics 4.0 is packed with the hope of a machine-driven, egalitarian world run by humanity-loving managers and technologists. We should redefine the challenge of automation as an opportunity to increase: to combine smart humans with smart machines to obtain the best overall.
Now, instead of contemplating “Which tasks will be performed quickly by computers currently worked by humans?” I would instead ask optimistically” What unique feats can companies accomplish when they have better-thinking computers to help them? Or how can we avoid numbers of deaths with improved AI evacuation practices in a disaster-prone area? Or why can’t AI-driven e-schools be implemented in poverty-stricken areas?
Most companies are developing “cognitive” technologies intelligent appliances that automate elements of choice-making processes. Some do pilots exploring technology. Others focus on the principle of building a Consumer-AI-Controlled network. To have the task done, these networks use the concept of Personal AI agents who connect with other AI services. For just an AI-powered device, there will be no more manual efforts required to direct your daily activities.
Most data scientists now conclude that wholesale revisions are just too dangerous, and seek to split concepts into smaller bits instead. Every component is reviewed and then phased in the data flow with caution. I wouldn’t be surprised if any of these developments will make significant strides in the future. There is an element of ambiguity attached to them, but I am very confident about the future, unlike others.
Data Science has rapidly extended in the last ten years to include companies and organizations around the world. During its growth, the use of Big Data by Data Science was not only a scaling up of the data but included transitioning to new data management systems, researching and evaluating how data is processed.
Today, Data Science is becoming an essential part of scientific research and business. It involves technology, computer translation, robots, digital economy, voice recognition, and search engines. Data Science has grown to include the biological sciences, education and healthcare, clinical informatics, arts, and natural sciences in terms of study areas. Data Science is also influencing governments, economics, enterprises, and finance.
In conclusion, there’s no doubt that data science will keep evolving as industries keep changing. However, one point remains clear: there will always be demand for data scientists. There must be highly qualified specialists who can interpret it as long as data exists. The concerns for the future will be how much data will be accessible, where it will come from, and by then, what new analytical strategies will arise to bring us even higher insights.